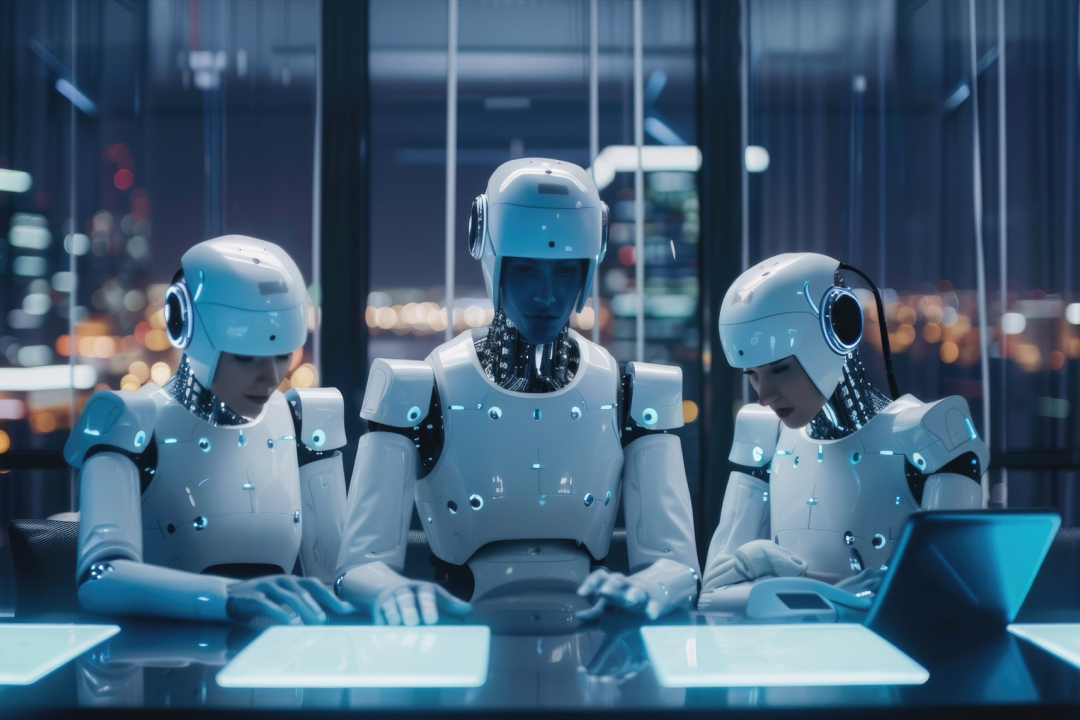
- August 22, 2024
- Abi Therala
- 0
Key Ingrediants to build successful GenAI team
Creating a successful Generative AI (GenAI) team involves more than just advanced technology. It requires strategic planning, the right talent, and a strong connection with market needs. Here’s a closer look at the key elements necessary for building a great GenAI company.
Vision and Strategy
A clear vision and strategic plan set the foundation for your company’s direction and success.
- Understanding Opportunities: Analyze industries where GenAI can have the most impact. For instance, in healthcare, AI can assist in diagnostics and personalized medicine. In finance, it can improve fraud detection and automate trading strategies. Understanding these opportunities helps you focus on areas with high potential.
- Product Fit: Develop solutions that address specific problems within these industries. For example, if targeting retail, you might create an AI tool for inventory management or personalized shopping experiences. Ensure your product solves a real problem and delivers clear benefits.
- Growth Plan: Create a roadmap for scaling your technology. This includes planning for increased data volume, expanding your team, and entering new markets. Having a strategy for scaling ensures that your company can handle growth without compromising performance.
Technology and Infrastructure
Robust technology and infrastructure are essential for delivering reliable and effective AI solutions.
- Advanced Models: Use state-of-the-art AI models such as GPT (Generative Pre-trained Transformer) for natural language tasks or GANs (Generative Adversarial Networks) for image generation. These models can handle complex tasks and provide high-quality results.
- Infrastructure: Invest in scalable cloud infrastructure to support large data processing and model training. Services like AWS, Google Cloud, or Azure offer tools and resources to manage and scale AI workloads effectively. Ensure your infrastructure can grow with your needs and handle peak loads.
- Data Management: Collect and preprocess data to train your models. This involves gathering relevant datasets, cleaning and labeling them, and ensuring data quality. High-quality data is crucial for training accurate and effective AI models. Implementing a robust data management strategy helps maintain data integrity and usability.
Talent and Team
Building a skilled and motivated team is critical for innovation and execution.
- Hire Experts: Recruit individuals with expertise in AI, machine learning, and data science. Look for candidates with experience in developing and deploying AI solutions, as well as a track record of solving complex problems.
- Ongoing Training: Encourage continuous learning and professional development. AI is a rapidly evolving field, so providing opportunities for your team to stay updated on the latest advancements helps maintain a competitive edge.
- Collaborative Culture: Foster a collaborative environment where team members from different disciplines—such as engineering, data science, and product management—work together. Effective collaboration leads to better problem-solving and innovation.
Customer Focus
Aligning your products with customer needs ensures relevance and satisfaction.
- Feedback Systems: Implement mechanisms to gather and analyze feedback from users. This can include surveys, user testing, and direct interactions. Use this feedback to make iterative improvements to your products.
- Customization: Offer solutions that can be tailored to individual customer needs. For instance, provide configurable AI tools that can be adapted to different business processes or user preferences.
- User-Friendly Design: Design your AI tools to be intuitive and easy to use. A user-friendly interface enhances the customer experience and ensures that users can effectively leverage the AI capabilities.
Ethics and Responsibility
Develop and deploy AI responsibly to build trust and avoid negative impacts.
- Ethical Standards: Adhere to ethical principles in AI development, such as transparency, fairness, and accountability. This includes being open about how AI models make decisions and ensuring they are used in ways that are ethical and beneficial.
- Bias Prevention: Actively work to identify and address biases in your AI models. This involves regularly testing for biases, diversifying training data, and implementing techniques to mitigate bias.
- Legal Compliance: Stay informed about laws and regulations related to AI. This includes data protection laws (like GDPR), industry-specific regulations, and emerging guidelines for AI ethics.
Partnerships and Networking
Building relationships with other organizations can drive growth and innovation.
- Academic Ties: Partner with universities and research institutions to collaborate on cutting-edge AI research. These partnerships can provide access to new technologies and insights that enhance your offerings.
- Industry Connections: Engage with other companies and industry groups to share knowledge and develop standards. Collaborating with other organizations can lead to new opportunities and improve industry practices.
- Open Source: Contribute to and leverage open-source projects. This not only helps foster innovation but also builds credibility and allows you to benefit from community-driven improvements.
Business Model
A sustainable and scalable business model ensures long-term success.
- Subscription Models: Offer AI services through subscription plans. This provides a steady revenue stream and allows customers to access AI tools on an ongoing basis.
- AI-as-a-Service: Provide AI capabilities via cloud platforms, enabling customers to use AI without investing in their own infrastructure. This model allows for flexible scaling and can attract a broader customer base.
- Data Utilization: Explore ways to monetize data, such as offering analytics services or selling insights derived from data. Ensure that data monetization strategies comply with privacy regulations and ethical standards.
Innovation and Adaptability
Staying ahead in the AI field requires constant innovation and flexibility.
- Invest in R&D: Allocate resources to research and development to explore new AI technologies and applications. This investment helps you stay at the forefront of the industry and drive technological advancements.
- Be Agile: Maintain the ability to pivot quickly in response to new trends, market demands, or technological developments. Agile practices enable you to adapt and capitalize on emerging opportunities.
- Customer-Inspired Ideas: Use insights from customer interactions to guide product development. Understanding customer pain points and preferences helps you create relevant and effective solutions.
Global Reach
Expanding globally opens up new markets and opportunities.
- Expand Internationally: Identify and enter new markets to grow your customer base. Tailor your products and marketing strategies to fit different regions and industries.
- Adapt for Local Needs: Customize your AI solutions to accommodate different languages, cultural norms, and regulatory requirements. This ensures that your products are effective and relevant in diverse markets.
- Regulatory Awareness: Be aware of international regulations affecting AI. Compliance with global standards helps you avoid legal issues and builds trust with international customers.
Conclusion
Building a successful GenAI company involves a strategic mix of technology, talent, and customer focus. By addressing these key areas, companies can create impactful AI solutions, drive innovation, and achieve long-term success.