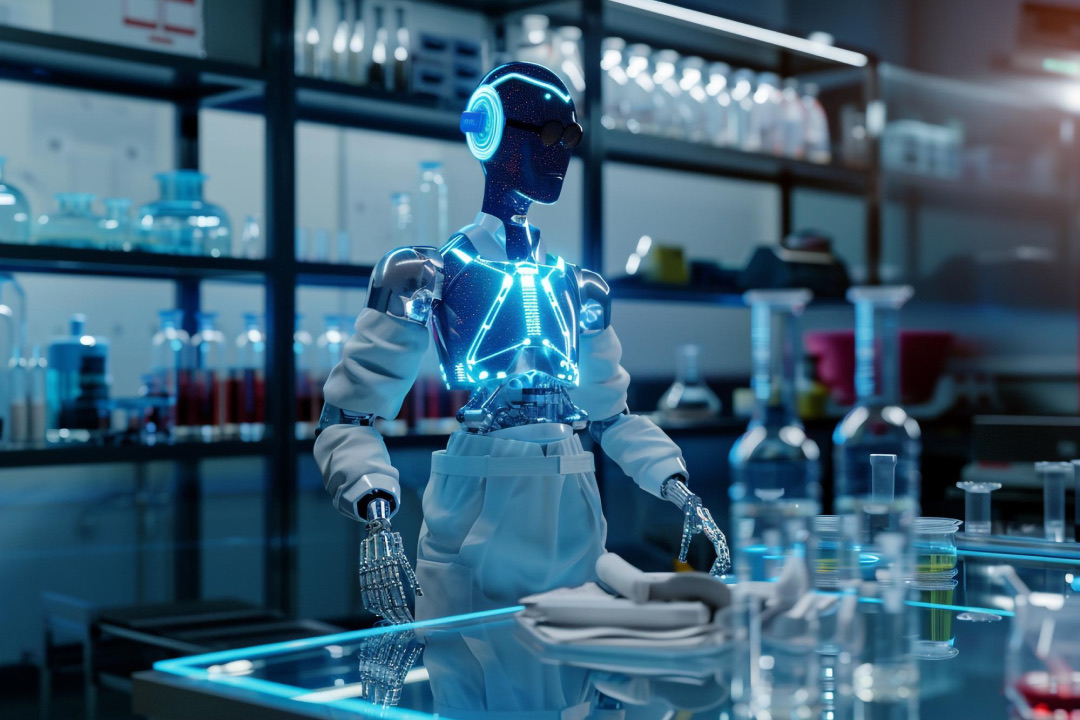
- October 21, 2024
- Abishek Terala
- 0
How Generative AI is Accelerating Drug Discovery
Drug discovery and development have long been plagued by inefficiencies, high costs, and extensive timelines. The pharmaceutical industry faces immense challenges in bringing new drugs to market, typically requiring 10-15 years and costs exceeding $2.3 billion per drug. The process, marked by numerous stages of experimentation, testing, and regulatory approval, is expensive and fraught with high failure rates.
In the face of these challenges, Generative AI (Gen AI) offers a revolutionary shift in how drugs are discovered, tested, and brought to market. By leveraging advanced algorithms to simulate chemical compounds, predict molecular interactions, and optimize clinical trials, Gen AI has the potential to dramatically cut down costs and accelerate timelines.
The Traditional vs. AI-Driven Drug Discovery Process
The differences between the traditional drug discovery methods and those driven by AI-based approaches are profound. Gen AI enables an iterative, data-driven process that can generate new compounds, optimize screening, and predict outcomes with far greater speed and precision than traditional methodologies, which are slower and more labor-intensive.
Benefits of Generative AI in Drug Discovery
Speeding Up Molecular and Compound Generation
One of the standout benefits of Gen AI is its ability to quickly design and evaluate new molecules. Traditional approaches involve years of manual work to test chemical compounds in laboratories, but AI-driven models, like those from Insilico Medicine and BenevolentAI, can simulate the properties of potential drug molecules and reduce the timeline to mere months.
Generative AI can process massive chemical datasets, predict how molecular compounds will behave, and optimize them for specific therapeutic needs. In an example from Adaptyv Bio, researchers generated protein designs using Gen AI, combined with robotics, to create optimized compounds in a matter of weeks. This process allows AI-driven models to explore chemical spaces that were previously inaccessible, speeding up the discovery phase by 3-4x.
Cost Reduction in Drug Development
Pharmaceutical R&D expenditures have skyrocketed, reaching over $6.16 billion per drug, according to some estimates. Generative AI has the potential to reduce these costs to one-tenth of the traditional methods by automating early drug discovery and screening processes. For instance, Insilico Medicine advanced a drug from conception to clinical trials at only a fraction of the traditional cost by leveraging AI to optimize molecule generation.
Beyond molecular design, AI helps reduce the time and resources spent on unsuccessful candidates. By predicting which drugs will fail before reaching costly clinical trials, companies can allocate their funds more efficiently, reducing R&D waste.
Improved Precision and Personalization
One of the most transformative aspects of Generative AI in drug discovery is its ability to enable highly personalized treatments. By integrating patient data such as genetic markers and biomarkers, Gen AI models can develop drugs tailored to specific populations or even individuals. The ability to screen compounds based on a patient’s unique genetic makeup means that personalized medicine is no longer just an ambition but a rapidly approaching reality.
AI-driven systems, like PandaOmics by Insilico, are already being used to identify biomarkers associated with diseases such as gallbladder cancer and alopecia. This capability ensures that drugs target the right mechanisms in the right patients, drastically improving the chances of success and enhancing patient outcomes.
Predicting Clinical Trial Outcomes and Reducing Failure Rates
An estimated 90% of drug candidates fail in clinical trials, leading to vast financial losses. Generative AI can mitigate this risk by predicting the success of drug candidates based on simulations, historical trial data, and patient characteristics. AI models, such as Insilico’s inClinico, demonstrated 79% accuracy in predicting clinical trial outcomes, a game-changer for the industry.
These predictions allow researchers to refine trial designs before launching them, thereby reducing failure rates and expediting the path to approval. Gen AI models can also support adaptive trial designs, dynamically adjusting parameters as new data emerges, further increasing the likelihood of favorable outcomes.
Expanding the Impact of Generative AI in Drug Discovery
AI-Powered Molecule and Compound generation
In the realm of drug discovery, AI models trained on vast chemical datasets can generate novel molecules that would take years to discover through traditional means. These molecules are then optimized to meet specific therapeutic goals, balancing potency, safety, and synthetic feasibility.
For instance, Insilico Medicine used AI to generate the molecule ISM6331, targeting advanced solid tumors. The AI system explored over 6,000 molecular possibilities and selected a few for preclinical testing, drastically reducing the time needed to identify promising drug candidates.
Impact Metrics:
- Speed of Discovery: Reduced time from years to months.
- Efficiency: Thousands of molecules screened in silico.
- Cost Reduction: Lowered R&D expenses by reducing laboratory experiments.
Biomarker Identification for Personalized Treatments
Gen AI excels in analyzing large genomic and proteomic datasets to identify disease-specific biomarkers, which can then be used to develop personalized drugs. AI models sift through millions of data points to identify biomarkers that can predict drug responses or disease progression. For example, Insilico Medicine’s PandaOmics identified biomarkers for diseases like gallbladder cancer.
Impact Metrics:
- Improved Patient Stratification: Biomarkers ensure better matching of patients to treatments.
- Success Rates: Increased likelihood of trial success due to targeted therapies.
Optimizing Drug-Target Interaction Studies
Gen AI allows researchers to simulate how drugs will interact with their target proteins. By mapping the binding affinity between a drug and its target, AI can help researchers avoid dead ends and focus on the most promising candidates.
MIT researchers, for instance, developed a Gen AI model that screened over 100 million compounds in just one day to identify 19 promising drug-target pairs. Of these, 12 were later validated as having strong binding potential, drastically improving the efficiency of drug-target interaction studies.
Impact Metrics:
- Screening Speed: Billions of molecules evaluated in days rather than months.
- Enhanced Success Rates: Greater precision in drug-target pairing.
Accelerating Drug Repurposing and Combination Therapies
AI models are also being used to explore new therapeutic applications for existing drugs, a much faster and cheaper process than developing drugs from scratch. Gen AI tools can predict how combinations of existing drugs may treat complex diseases. For instance, researchers at IBM used AI to identify rasagiline, a Parkinson’s drug, as a candidate for treating dementia.
Impact Metrics:
- Time to Market: Repurposed drugs can reach market faster.
- Lower Development Costs: Saves billions in R&D expenses by bypassing early-stage research.
Challenges of Implementing Generative AI in Drug Discovery
While Gen AI offers unparalleled opportunities, several challenges remain:
- Model Explainability
Researchers often face difficulties in understanding how AI models reach certain conclusions, creating a “black box” problem. For drug discovery, explainability is critical for regulatory approval and scientific validation. - Model Hallucinations and Inaccuracies
Gen AI models may sometimes generate plausible but incorrect predictions, such as molecules that cannot be synthesized or interactions that lead to toxicity. Careful validation and human oversight are essential. - Data Bias
If trained on biased datasets, AI models may produce skewed results. Ensuring diverse and comprehensive datasets is critical for producing reliable outputs. - High Computational Costs
Running large-scale AI models requires significant computational resources, driving up costs. While cloud-based solutions help mitigate this, it remains a barrier to widespread adoption.
A New Era for Pharma and Healthcare
Generative AI is revolutionizing the pharmaceutical industry by accelerating drug discovery, reducing costs, and enhancing precision. From generating novel compounds to predicting clinical trial outcomes, AI is transforming how we develop drugs, enabling faster and more effective treatments for patients worldwide. While challenges remain, the future of drug discovery looks promising with the continued integration of AI into every facet of the process.
Frequently Asked Questions (FAQs)
- How is Generative AI used in drug discovery?
It automates molecule generation, predicts interactions, and enhances clinical trials, significantly reducing time and costs. - What are the main benefits of Gen AI in drug development?
Faster drug discovery, reduced R&D costs, personalized treatments, and higher success rates in clinical trials. - Can AI help with drug repurposing?
Yes, AI can find new uses for existing drugs, speeding up the development process and reducing costs. - What are the challenges of using AI in pharma?
AI can predict success rates, optimize trial designs, and enhance patient recruitment, making trials faster and more efficient.
Sources:
- Generative AI in Pharmaceuticals: Accelerating Drug Development and Clinical Trials
- Reshaping Drug Discovery: How Generative AI and Digital Twins are Accelerating Innovation
- GAIP: Applications of Generative AI in Drug Discovery
- Moving from Hype to Reality: The Role of Gen AI in Pharma