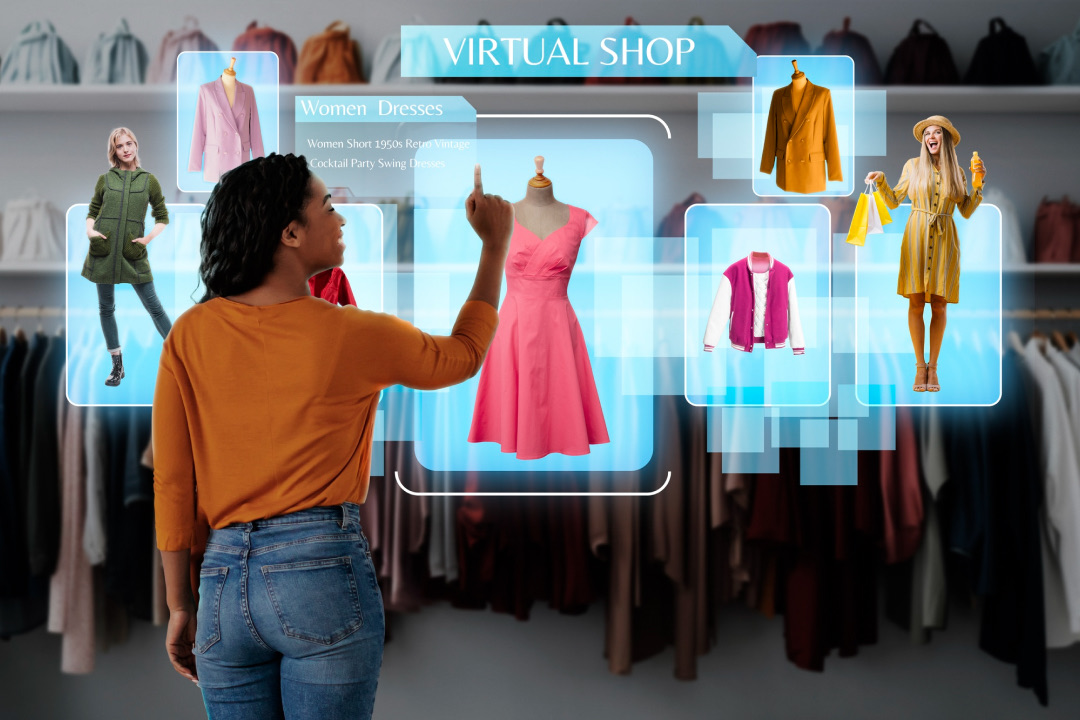
- October 23, 2024
- Abishek Terala
- 0
Generative AI in Fashion: Revolutionizing Design, Marketing, and Sustainability
The fashion industry has always thrived on creativity, innovation, and the ability to predict and set trends. Today, generative AI is transforming the way fashion is designed, manufactured, marketed, and sold, creating a shift that is as much about artistic evolution as it is about operational efficiency. By leveraging advanced algorithms, generative AI allows designers to push creative boundaries while giving brands the tools to optimize production and marketing strategies
How is generative AI changing the face of fashion?
The fashion industry faces intense competition, fast-changing trends, and increasing pressure for sustainable production. As brands strive to cater to the diverse and dynamic preferences of their global customer base, AI-driven solutions are emerging as indispensable tools. Generative AI in particular addresses key challenges:
- Trend Prediction and Forecasting: Fashion trends are volatile. Generative AI uses vast datasets from social media, retail patterns, and influencer behaviors to predict upcoming trends and customer preferences.
- Sustainability: With increasing consumer demands for eco-friendly products, AI optimizes production processes and material selection, reducing waste and improving the environmental footprint.
- Personalization: Today’s shoppers demand personalized experiences, from product recommendations to custom designs, and AI excels at making each shopping journey unique.
How GANs Transform Fashion Design
Generative Adversarial Networks (GANs) play a pivotal role in reshaping the fashion industry by generating unique designs, realistic virtual models, and personalized clothing items. GANs consist of two neural networks—a Generator and a Discriminator—that work together to create new outputs and refine their quality through an adversarial process.
- Dataset: The process starts with vast datasets that include fashion-related information such as clothing images, textures, color palettes, and patterns. These datasets often contain millions of labeled images, ranging from runway shots to catalog photos, annotated with information like style, fabric, and fit. AI models learn from this data to understand fashion aesthetics and consumer preferences.
- Wearer Image: To customize fashion for individual users, the system can take a wearer image—either a body scan or a simple photo uploaded by the customer. This image provides crucial details such as body shape, size, and fit preferences. The wearer image acts as a template onto which the AI-generated fashion designs will be virtually applied.
- Generator: The Generator neural network is the creative powerhouse in the GAN model. It starts with the dataset and wearer image inputs to generate realistic clothing designs or styles that fit the wearer’s body. In this step, the Generator can create various outputs—such as dresses, shirts, or entire outfits—based on input parameters like style, season, or trend data.
The Generator takes inspiration from historical designs in the dataset but adds new, unique elements, ensuring each output is original and tailored. For example, if the input is a summer dress with floral patterns, the generator will use those cues to produce a range of stylistic variations that blend creativity with real-world practicality. - Controller: The Controller is responsible for refining the process. It acts as a middle layer that regulates how the Generator operates based on specific rules or guidelines set by the designer or brand. The Controller can ensure the generated designs adhere to a brand’s aesthetic or sustainability guidelines, making sure outputs are marketable and aligned with consumer expectations. For example, it could ensure that a generated design uses only sustainable materials or fits a specific price range.
In fashion, the Controller may also tweak the generated outputs based on real-time consumer data, ensuring designs are relevant to the current market trends and seasonal demands. - Discriminator: Once the Generator creates a design, the Discriminator evaluates its authenticity. It compares the generated design with real-world fashion items from the dataset to decide whether the output looks “real” or if it needs further refinement. The Discriminator acts like a fashion critic, constantly challenging the Generator to produce more realistic and appealing designs.
In the context of virtual fashion models, the Discriminator ensures that AI-generated outfits appear lifelike when applied to the wearer image. It scrutinizes factors such as how the fabric drapes on the body, the accuracy of color rendering, and how well the design fits the wearer’s dimensions.
Source: Neural Comput & Applic 33, 8445–8457
Top 5 Use Cases of Generative AI in Fashion
- AI-Assisted Creative Designing
AI offers fashion designers a powerful toolkit to generate and refine designs more quickly than ever before. By providing vast creative inputs and enabling real-time iteration, generative design ensures that both established and aspiring designers can unleash innovative collections within minutes.
Example: Tools like DALL-E 3 can create highly stylized and artistic designs from mere textual descriptions, making the design process faster and more efficient. Designers can describe the type of outfit they want (e.g., “A futuristic gown with geometric patterns”) and receive multiple AI-generated designs instantly. - Transforming Fashion Sketches into Full Designs
Generative AI tools have the unique ability to transform simple, hand-drawn sketches into fully realized, colored images. Designers no longer need to manually color or envision final textures, as AI models can handle this task with precision.
Scientific Impact: Pix2Pix algorithms can instantly convert grayscale fashion sketches into fully colored, lifelike renderings, allowing designers to visualize their concepts in different hues, fabrics, and lighting. This allows experimentation with minimal resource expenditure. - AI-Generated Virtual Fashion Models
Virtual fashion models generated by AI help brands represent a diverse range of body types, ethnicities, and styles, enabling customers to better visualize how garments will look on them.
Example: Japanese startup DataGrid uses Generative Adversarial Networks (GANs) to produce hyper-realistic virtual models. These AI-generated models offer flexibility in terms of size, appearance, and even motion, providing a completely customizable virtual fashion show experience.
Virtual try-ons are another use case where AI plays a vital role. By integrating Augmented Reality (AR), customers can use their smartphones to virtually “try on” outfits, reducing the need for physical store visits or returns. - AI in Marketing and Trend Prediction
One of the strongest applications of AI in fashion is in marketing and trend analysis. Natural Language Processing (NLP) and Machine Learning (ML) algorithms scour data from social media platforms, runway events, and consumer feedback to predict upcoming trends.
Example: Retail giant Zara leverages AI-driven trend forecasting to plan its seasonal collections, adjusting its inventory and marketing strategies in real time based on these insights.
AI can also automate personalized marketing campaigns, making sure consumers receive the latest fashion suggestions tailored to their preferences. AI-generated emails, targeted social media ads, and product recommendations based on browsing history can drive engagement and conversions. - Sustainability Through Generative AI
Generative AI contributes to sustainability in fashion by optimizing material use, reducing fabric waste, and improving production efficiency. AI-driven material selection platforms analyze the environmental impact of different fabrics and suggest eco-friendly alternatives.
Example: Tools like CircularID help designers and brands track the entire lifecycle of a product, from design and manufacturing to consumer use and eventual recycling or disposal. AI ensures transparency, allowing companies to uphold sustainable values while maintaining profitability.
Generative AI also improves lean manufacturing practices by ensuring brands only produce as much as they need, cutting down on excess inventory and waste.
Challenges and Ethical Concerns in AI Fashion
As promising as generative AI is, it comes with its share of challenges:
- Intellectual Property Issues: Who owns the designs created by an AI model? Is it the fashion brand, the designer, or the software developer? This question of authorship needs resolution as AI-generated designs become more common.
- Loss of Human Creativity: Some fear that AI may reduce the role of human creativity in fashion. However, most experts agree that AI is a tool to augment creativity, not replace it.
- Bias in AI Models: AI systems trained on biased datasets might produce designs that lack inclusivity, whether in terms of body representation, ethnicity, or style diversity. Careful curation of data is essential to avoid reinforcing stereotypes.
The Future of AI in Fashion
As generative AI continues to evolve, it will play a more significant role in every aspect of fashion, from design to marketing to sustainability. Some exciting trends include:
- AI-Generated Custom Fashion: In the near future, consumers could have entire collections customized for them, from fabric choices to color schemes, based on their personal style preferences.
- AI-Powered Sustainable Fashion: AI will help brands fully adopt circular fashion practices, where clothes are designed to be recycled, repurposed, or biodegraded.
- Integration with Blockchain: By combining AI with blockchain, brands can track the complete lifecycle of a garment, from raw material sourcing to post-purchase recycling, ensuring transparency in sustainability efforts.
Conclusion
Generative AI is transforming fashion into a more sustainable, efficient, and creatively diverse industry. By automating repetitive tasks, generating innovative designs, and optimizing resources, AI empowers fashion brands to be more agile, consumer-centric, and eco-friendly. The future of fashion will undoubtedly be shaped by these innovations, leading to a more personalized and sustainable global fashion ecosystem.
Frequently Asked Questions (FAQs)
- How is generative AI revolutionizing the fashion industry?
Generative AI is transforming fashion by streamlining the design process, predicting trends, and enhancing personalization. It allows designers to generate new, innovative clothing concepts quickly, while brands can use AI to optimize marketing and production, making fashion more sustainable and efficient. - How does generative AI improve sustainability in fashion?
Generative AI enhances sustainability by optimizing material use, minimizing fabric waste, and supporting eco-friendly design choices. AI-driven tools also help brands manage lean manufacturing, producing only what is needed, thus reducing overstock and environmental impact. - What role do GANs play in fashion design?
Generative Adversarial Networks (GANs) are crucial in fashion for creating unique designs, generating virtual models, and enhancing product personalization. GANs work through a generator and discriminator network, producing new designs and refining their realism based on existing data. - Can generative AI predict fashion trends?
Yes, generative AI leverages large datasets from social media, retail patterns, and consumer behaviors to accurately predict upcoming fashion trends. This allows brands to stay ahead of the curve and tailor their collections to meet consumer preferences. - How does AI-driven personalization benefit fashion consumers?
AI-driven personalization tailors fashion recommendations to individual customers based on their browsing history, preferences, and style. This creates a more engaging and customized shopping experience, driving higher satisfaction and loyalty.
References:
- McKinsey Report on AI in Fashion
- DataGrid Virtual Model Technologies
- ClothingGAN and StyleGAN AI Applications in Fashion