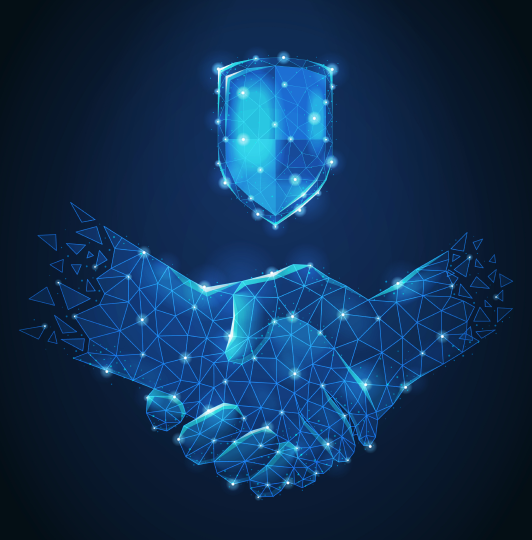
- March 15, 2024
- Abishek Terala
- 0
Bridging Trust Gaps in AI Implementation
Artificial intelligence (AI) is rapidly transforming our world, impacting everything from healthcare and finance to entertainment and transportation. However, alongside its undeniable potential lies a growing concern: trust. According to a PwC report, over half of global executives believe a lack of trust in AI is a significant barrier to adoption. This highlights the critical need to build trust in AI for its successful and ethical integration into society.
Why Trust in AI Matters
Earning public trust is essential for AI to achieve its full potential. Here’s why:
Fairness and Bias
AI algorithms can perpetuate societal biases if trained on biased datasets. This can lead to discriminatory outcomes, such as loan denials or biased hiring practices. Expanding fairness efforts includes conducting thorough bias assessments, employing fairness-aware machine learning algorithms, and fostering collaboration among diverse teams to identify and mitigate biases effectively.
Transparency and Explainability
Often referred to as “black boxes,” AI systems can be difficult to understand. Without transparency into how AI arrives at decisions, it’s challenging to hold them accountable or identify potential errors. Enhancing transparency involves providing clear insights into decision-making processes using interpretable machine learning models and fostering open dialogue between developers, ethicists, and domain experts.
Privacy and Security
AI systems often rely on vast amounts of personal data. Data breaches or misuse of this data can have serious consequences for individuals. Strengthening privacy and security entails implementing robust data protection measures, such as encryption and access controls, and promoting transparent data handling practices through clear consent mechanisms and privacy policies.
Building Trustworthy AI
Several key principles can guide the development and deployment of trustworthy AI:
Transparency
Explain how AI systems work and the rationale behind their decisions. This fosters user understanding and builds confidence. Efforts include articulating decision-making processes using interpretable machine learning models and promoting open dialogue between developers and users.
Fairness
Actively identify and mitigate bias in datasets and algorithms. Ensure diverse teams develop and test AI systems to consider different perspectives. Strategies involve conducting bias assessments, employing fairness-aware machine learning algorithms, and fostering collaboration among diverse teams.
Accountability
Establish clear lines of accountability for the development, deployment, and outcomes of AI systems. This includes defining roles and responsibilities across the AI lifecycle, implementing governance structures, and promoting transparency through public disclosures of AI practices and outcomes.
Privacy
Implement robust data security measures and obtain clear user consent for data collection and usage. This includes enforcing encryption and access controls, promoting transparent data handling practices through clear consent mechanisms and privacy policies, and fostering user trust through transparent data handling practices.
Security
Safeguard AI systems from manipulation or hacking to ensure reliable results. Strategies include integrating security-by-design principles into AI system development, implementing mechanisms for traceability and auditability, and fostering a culture of ethical responsibility and accountability within organizations.
Regulations and Standards
As AI continues to evolve, so too do efforts to regulate its development and use. Governments and industry bodies are working on creating frameworks to ensure responsible AI practices. Examples include the European Union’s General Data Protection Regulation (GDPR) and the Algorithmic Justice League’s Standards for Equitable Algorithms. While regulations are still evolving, they represent a vital step towards building trust in AI.
The Role of Individuals
Public trust in AI isn’t solely the responsibility of developers and regulators. Individuals also have a role to play:
Staying Informed: Educate yourself about how AI works and the potential risks involved.
Demand Transparency: Ask questions about the AI systems you interact with. Supporting
Ethical AI: Advocate for responsible development and deployment of AI technologies.
The Future of AI Trust
Building trust in AI is an ongoing process. By working together – developers, policymakers, and the public – we can ensure AI is a force for good, driving innovation and progress while safeguarding our values and privacy.
Human oversight, crucial in ensuring ethical and responsible AI use, emphasizes that AI should not replace human judgment, particularly in critical decision-making processes. Equally essential is education and training, which equip individuals with the necessary knowledge and skills to understand and interact with AI responsibly. Open discussions about AI’s potential benefits and drawbacks facilitated through public engagement, are vital for building public trust and ensuring responsible development. By prioritizing these principles, navigating the AI trust landscape becomes a collaborative effort, paving the way for a future where AI serves humanity and empowers positive change.