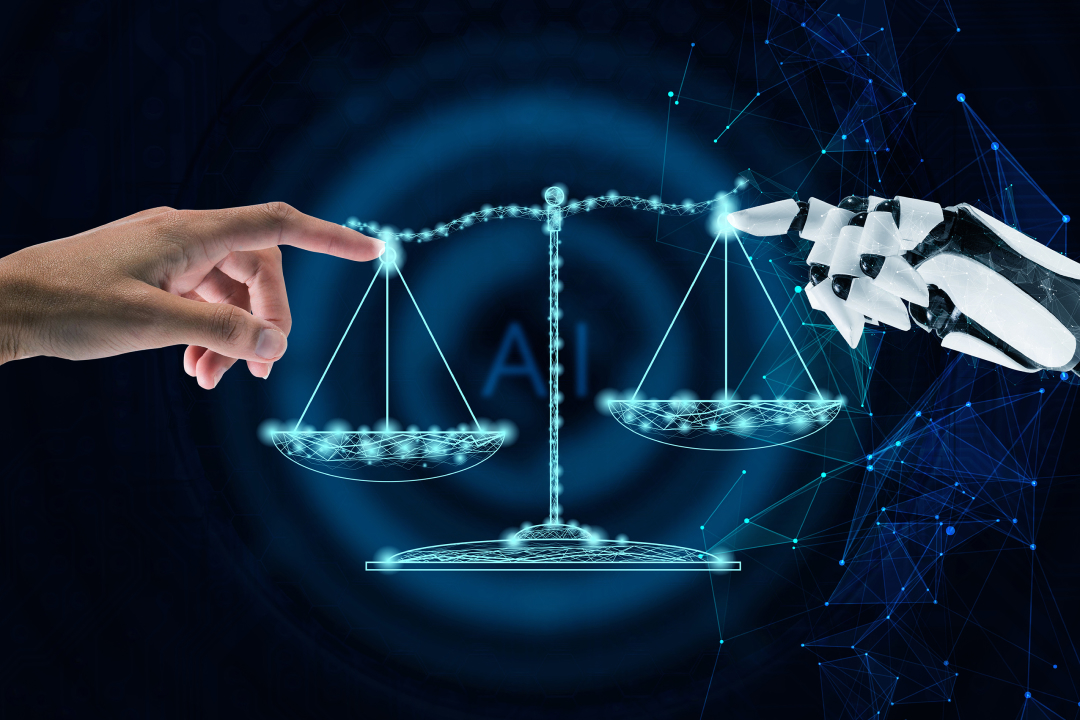
- June 21, 2024
- Abi Therala
- 0
Ethical considerations of Generative AI
For centuries, the vision of creating a perfect being has driven human innovation, culminating in the development of models that emulate human actions. This ambition has given rise to Generative AI, a transformative force with the potential to revolutionize every aspect of our lives. Generative AI amplifies human creativity and excels in real-time applications, impacting diverse domains such as education, customer support, marketing, health, and even space exploration. However, with its rapid advancement, the ethical considerations of Generative AI cannot be overlooked.
Unleashing Creative Potential
Artistic Exploration:
- Breaking Creative Blocks: Generative AI serves as a powerful tool for artists, musicians, and writers to overcome creative hurdles. For example, musicians can generate multi-genre compositions, while visual artists can engage in virtual collaborations that push the boundaries of traditional art forms. This collaboration between human creativity and AI can lead to unprecedented innovations in the arts, offering new ways to express and experience creativity.
- Personalized Content Creation: The ability of Generative AI to quickly and originally create content offers significant advantages for businesses. Marketing campaigns, social media posts, and other content can be tailored to specific audiences, enhancing engagement and effectiveness. This speed and originality not only streamline content production but also allow businesses to stay relevant in fast-paced digital landscapes.
Scientific Discovery:
- Drug Discovery: In the realm of pharmaceuticals, AI algorithms can analyze vast datasets of chemical and physical properties to generate new molecules. This capability accelerates drug development by predicting molecular behaviour in biological contexts, thereby reducing both the time and cost associated with traditional drug discovery processes. This can lead to more rapid advancements in medicine and the development of treatments for various diseases.
- Planetary Exploration: Generative AI plays a crucial role in space science by aiding the search for habitable exoplanets. Machine learning models can analyze planetary atmospheres to predict the presence of essential elements like water and methane, indicating potential signs of life. This accelerates our understanding of the universe and supports missions aimed at discovering new worlds.
Revolutionizing Education:
- Personalized Learning: Generative AI can transform education by providing personalized learning experiences. By analyzing vast amounts of data, AI can identify each student’s unique learning style, preferences, and strengths. This allows educators to create customized educational content that addresses individual needs, improving student engagement and learning outcomes.
- Accessible Education: AI can also make education more inclusive. Features such as text-to-speech, speech-to-text, and automatic captioning ensure that learning materials are accessible to people with disabilities. This philanthropic approach to education democratizes access to knowledge, helping to bridge educational gaps and promote lifelong learning.
Augmenting Human Capabilities:
- Prosthetic Limb Design: Generative AI significantly enhances the development of prosthetic limbs. By analyzing body signals (EMG), AI can create active prosthetics that offer improved functionality and comfort. This leads to better mobility for users and reduces the time required for design iterations, ultimately improving the quality of life for individuals with limb differences.
- Generative Design: In product development, AI can automate the design process, generating multiple iterations that refine style, functionality, and efficiency. This iterative process allows for the rapid prototyping of innovative solutions, paving the way for more efficient and creative product designs in various industries.
These examples illustrate the expansive potential of Generative AI to drive innovation across multiple domains. However, its ethical implications demand vigilant scrutiny. As Generative AI evolves, it is crucial to balance its benefits with ethical considerations to ensure that its advancements contribute positively to society.
The Digital Identity Crisis
The proliferation of open-source language models (LLMs) and rogue AI bots threatens to undermine human control over the internet. These models can generate convincing deepfakes, creating ethical dilemmas and security concerns. Deepfakes can mimic voices and images with alarming accuracy, leading to scams, blackmail, and fraud. Celebrities and ordinary individuals alike are vulnerable to these attacks, which can cause significant personal and professional harm.
The ability of LLMs to generate fake engagement on social media platforms exacerbates the issue, shaping political narratives and influencing public perception through misinformation. This manipulation undermines the integrity of democratic processes and erodes trust in the media.
To counteract these threats, various measures are being implemented:
- Social Media Regulations: Platforms like Twitter Blue have introduced paid verification to increase transparency. Meta has mandated disclosure policies for AI-modified content, particularly in political, social, and election-related advertisements. These measures aim to reduce misinformation and enhance accountability.
- Technological Solutions: Advanced technologies like digital watermarking and distributed ledgers help verify the authenticity of digital content. Providing metadata access to video publishers can further mitigate the misuse of deepfakes and other malicious activities, ensuring greater trust in online information.
Breach in the Cognitive Domain
Generative AI’s ability to reconstruct natural images from recorded brain activity using Deep Neural Networks raises significant ethical concerns. This technology can be misused by malicious actors to manipulate users psychologically. Tools like WormGPT and FraudGPT can exploit vulnerabilities in applications like ChatGPT and DALL-E2, generating harmful content intended to provoke fear, anger, or other strong emotional reactions.
To combat these threats, researchers have developed Decoded Neurofeedback-based Generative Adversarial Networks (DecNefGAN). This adversarial solution combines AI with brain imaging (fMRI) in a closed-loop system to counter AI-generated stimuli. By altering brain patterns in response to harmful content, DecNefGAN can safeguard cognitive vulnerabilities. This technology has potential applications in treating PTSD, enhancing personalized AI training, and promoting accelerated learning.
Violation of Data Privacy
Generative AI poses significant risks to data privacy, particularly through exfiltration attacks. These attacks can compromise sensitive information used to train AI models, threatening an organization’s legal and intellectual property rights. In some cases, this information can be leaked to the public or competitors, leading to misinformation and exploitation.
To address these privacy concerns, several strategies can be employed:
- Federated Learning: This approach ensures data privacy by training AI models across decentralized devices without sharing raw data.
- Differential Privacy: Techniques that add noise to datasets protect individual data points, ensuring privacy during AI training.
- Data Security Measures: Anonymizing data, using secure enclaves, and adhering to regulations like GDPR and CCPA can mitigate risks. Regular updates to data security algorithms are essential to stay ahead of potential threats.
Bias Amplification
Generative AI, despite its potential, can exacerbate existing biases, particularly in talent management. Biases in traditional hiring practices can be perpetuated by AI models, leading to unfair outcomes. For instance, resume screening algorithms might overlook qualified candidates based on keyword choices or formatting, while AI-powered leadership programs might favor certain demographics.
To counteract these biases, several approaches are recommended:
- Human-in-the-Loop: Incorporating human judgment in AI decision-making processes ensures fairness and accountability.
- Data Augmentation and Scrubbing: Creating equitable datasets by augmenting and scrubbing data helps train unbiased AI models.
- Inclusive Tools: Modern applicant tracking systems (ATS) and resume builder tools should include inclusive language to remove unintentional barriers and promote diversity. These tools can help candidates reach a wider market, ensuring equal opportunities.
In conclusion, while Generative AI holds immense potential for innovation and progress, it also presents significant ethical challenges. Addressing these concerns through vigilant oversight, technological solutions, and regulatory measures is crucial to ensure that AI contributes positively to society. By balancing the benefits and risks, we can harness the power of Generative AI to create a better, more equitable future.