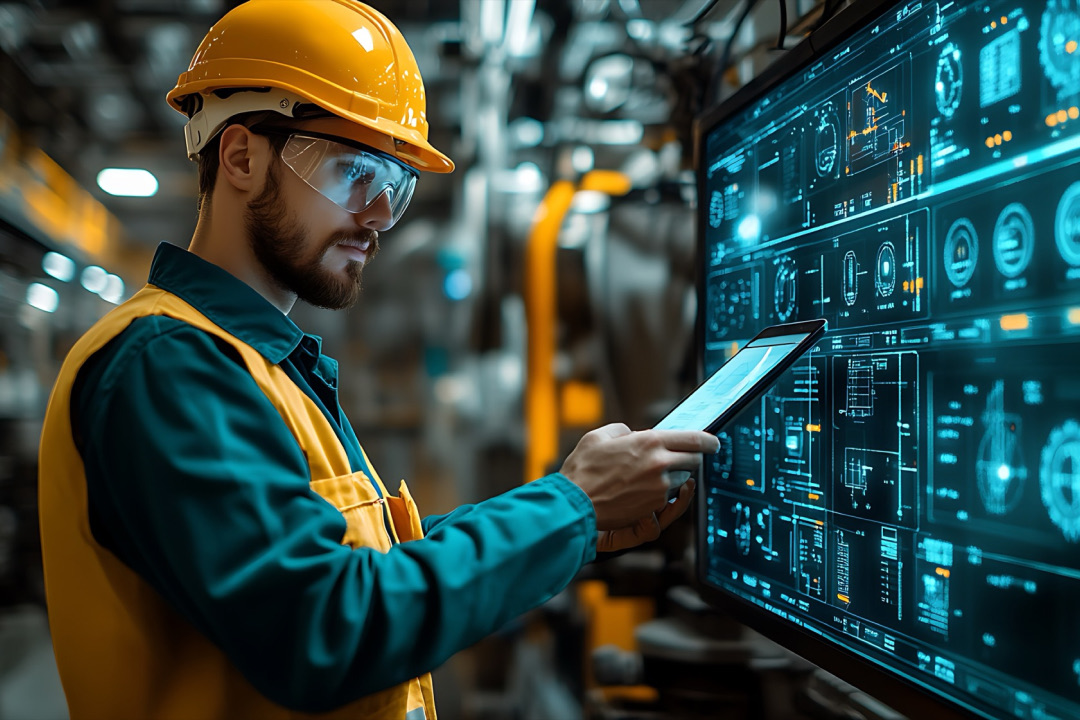
- October 29, 2024
- Abishek Terala
- 0
The Role of Generative AI in Predictive Maintenance
As businesses navigate increasingly complex supply chains, one critical goal remains constant: minimizing disruptions due to equipment failure. Predictive maintenance is central to achieving this goal, using advanced analytics to anticipate and prevent equipment failures. With the recent advent of Generative AI, predictive maintenance has reached new heights in precision, allowing organizations to simulate equipment performance, detect anomalies, and generate optimized maintenance schedules. This transformative approach empowers companies to keep their supply chains running smoothly and cost-effectively.
Predictive Maintenance Explained
Predictive maintenance is the proactive detection of potential equipment failures, enabled by analyzing sensor data and performance indicators. Unlike reactive maintenance, which involves fixing equipment after it fails, or preventive maintenance, which relies on routine checks, predictive maintenance uses real-time data and machine learning to schedule maintenance only when necessary. This targeted approach results in improved asset lifespan, optimized resource use, and minimized downtime.
Generative AI’s Game-Changing Role in Predictive Maintenance
Generative AI is not merely an enhancement to predictive maintenance but represents a breakthrough, capable of simulating and generating data for failure prediction. It helps create scenarios, run “what-if” simulations, and model equipment performance across varied conditions, extending predictive maintenance beyond traditional analysis.
Synthetic Data Generation for Improved Prediction
One challenge in predictive maintenance is the scarcity of failure data, especially when assets rarely malfunction. Generative AI overcomes this by creating synthetic data that represents potential wear patterns, stress conditions, or environmental changes. By training predictive models on this synthetic data, organizations can achieve higher accuracy and reliability in their maintenance strategies. This approach is especially useful in high-stakes industries such as manufacturing, where equipment must operate continuously and without fail.
Scenario Simulation for Real-Time Decision Support
With Generative AI, maintenance teams can simulate how equipment will behave under extreme conditions—such as increased loads, high speeds, or varying temperatures. These simulations allow maintenance teams to understand a machine’s thresholds and identify the best times for maintenance interventions. By creating “virtual stress tests,” Generative AI not only predicts failures but suggests optimized maintenance schedules, which enhances resource allocation and decision-making in real time.
Automated Maintenance Plan Generation
Generative AI can also help produce comprehensive maintenance plans that include specific repair instructions, parts requirements, and skill assessments for technicians. By drawing from both structured data (like past repair logs) and unstructured data (such as technician notes), it generates repair plans that align closely with the unique needs of each asset. These AI-driven plans ensure maintenance actions are both precise and timely, reducing wasteful practices while maximizing equipment uptime.
Real-World Use Case:
To illustrate, let’s examine a real-world example using Generative AI in the renewable energy sector, specifically wind turbines. The wind energy sector relies heavily on predictive maintenance to keep turbines functioning optimally, as unplanned downtimes can lead to significant revenue losses.
Wind Turbine Predictive Maintenance with Generative AI:
A common challenge with wind turbines is the difficulty of predicting when critical components, such as gearboxes or rotor blades, may fail, especially given the remote locations and varying weather conditions. Here’s how Generative AI optimizes predictive maintenance for wind turbines:
- Data Collection from IoT Sensors: Each wind turbine is equipped with IoT sensors that monitor parameters such as vibration, temperature, and rotor speed. This real-time data is critical for predictive maintenance.
- Synthetic Data Generation for Rare Failures: Because critical failures are rare but costly, historical data on these failures is limited. Generative AI fills this gap by simulating potential failure scenarios, creating synthetic data that reflects wear and tear under different environmental stresses.
- Simulating Stress Scenarios: Generative AI models simulate how each turbine component responds to varying wind speeds and directions, identifying points of weakness. For example, the AI model can forecast how prolonged high-speed winds affect blade stress and gearbox vibrations, generating maintenance schedules that are responsive to environmental conditions.
- Automated Maintenance Planning: Based on the predictions, Generative AI generates comprehensive maintenance plans, specifying which components require inspection, replacement, or lubrication. These plans are adapted for local conditions, such as seasonal weather, which impacts turbine performance and wear patterns.
By implementing this AI-driven maintenance approach, wind farms experience fewer breakdowns, improved energy output, and reduced operational costs. The success of Generative AI in such complex environments underscores its potential to transform predictive maintenance across various supply chain applications.
Digital Twins: The Generative AI-Powered Virtual Asset
Digital twins—virtual replicas of physical assets—further enhance predictive maintenance by allowing real-time simulation and monitoring. When integrated with Generative AI, digital twins dynamically learn from real-world data, adapting to operational changes. Here’s how digital twins work within predictive maintenance frameworks:
- Real-Time Monitoring: Digital twins receive continuous data from physical assets via IoT sensors, reflecting real-time conditions such as load, temperature, and wear.
- Predictive Scenario Testing: Maintenance teams use digital twins to test different failure scenarios virtually. For instance, they can simulate the effects of extreme operating conditions to see if any components are at risk, allowing for preventive actions before issues occur.
- Continuous Learning and Adaptation: Powered by Generative AI, digital twins evolve with each data input, constantly improving their accuracy. This continuous learning helps the AI model refine maintenance schedules, prioritize high-risk equipment, and adapt to changing operational conditions.
Key Advantages of Generative AI in Supply Chain Predictive Maintenance
- Reduction in Downtime and Costs: Generative AI optimizes maintenance schedules based on real-time and simulated data, reducing both unplanned downtimes and maintenance costs.
- Enhanced Decision-Making and Resource Allocation: By automating scenario testing and predictive modeling, Generative AI enables supply chains to allocate resources where they’re most needed.
- Increased Equipment Reliability and Safety: Continuous monitoring and real-time diagnostics provided by Generative AI significantly enhance asset reliability, ensuring equipment is consistently safe and compliant.
Implementation Challenges and Considerations
While the benefits are substantial, adopting Generative AI in predictive maintenance does come with challenges:
- Data Security: IoT sensors generate large volumes of data, requiring robust security measures to prevent breaches.
- Cost of Implementation: Initial investments in AI infrastructure and training can be high, and skilled professionals are essential to manage complex AI models effectively.
- Data Quality and Integration: Predictive models rely on consistent, high-quality data. Integrating IoT data with historical maintenance records can be challenging but is necessary to achieve high predictive accuracy.
The Future of Predictive Maintenance with Generative AI
The adoption of Generative AI in predictive maintenance is projected to expand, with advancements in edge computing and cloud technologies enabling faster data processing and more accurate predictions. As Generative AI continues to evolve, its application in predictive maintenance will drive new efficiencies, from self-optimizing systems to autonomous maintenance interventions. For organizations looking to build resilient, high-performance supply chains, Generative AI is set to become an indispensable asset.
FAQs
How does Generative AI enhance predictive maintenance?
Generative AI enables scenario simulation, synthetic data creation, and dynamic maintenance planning, helping predict and prevent equipment failures more accurately.
What is a digital twin, and how does it support predictive maintenance?
A digital twin is a virtual model of a physical asset that uses real-time data to simulate and monitor equipment performance, providing insights for proactive maintenance.
Why is synthetic data important in predictive maintenance?
Synthetic data generated by Generative AI compensates for gaps in historical data, especially for rare but critical failures, enhancing model accuracy.
What industries benefit most from Generative AI in predictive maintenance?
Sectors like manufacturing, energy, and logistics benefit due to their reliance on complex machinery and the high costs associated with equipment downtime.
What challenges exist in implementing Generative AI for predictive maintenance?
Challenges include ensuring data security, managing high initial implementation costs, and maintaining high-quality data integration for model training and analysis.