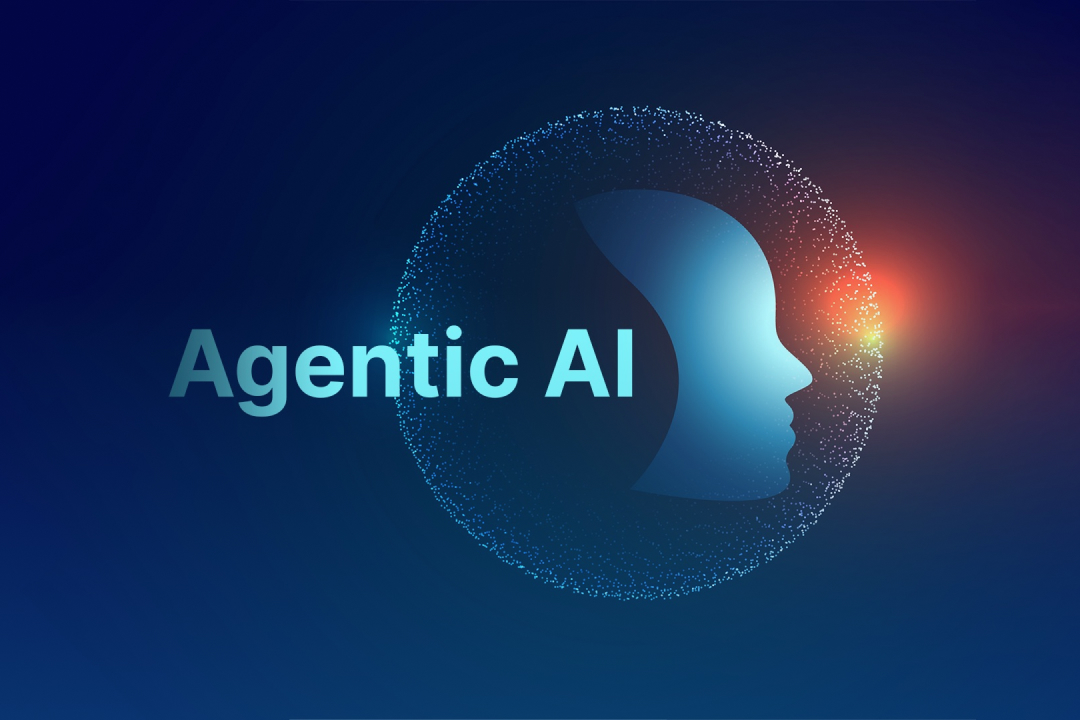
- April 23, 2025
- Abi Therala
- 0
What Is Agentic AI and Why Is Everyone Talking About It?
Agentic AI is quickly becoming one of the most exciting shifts in artificial intelligence. Unlike traditional AI models that follow fixed instructions, Agentic AI enables software agents to understand goals, make decisions, and act independently. As the digital economy demands smarter, more adaptive solutions, these autonomous agents are gaining attention for their ability to tackle complex tasks with human-like flexibility. This leap from passive automation to proactive, goal-driven intelligence is reshaping how AI is applied across industries and why it’s suddenly everywhere in the conversation.
How Does Agentic AI Actually Work?
Agentic AI systems are designed to operate like autonomous digital problem-solvers. Instead of waiting for step-by-step instructions, they can understand goals, plan how to achieve them, interact with people or tools, and adapt based on what happens. This makes them especially useful in open-ended, unpredictable environments—like managing financial strategies, automating research, or supporting complex customer service scenarios.
At the core, these systems follow a structured approach:
- Understanding the Goal: The process starts with the AI interpreting a user’s intent and translating it into a clear, actionable objective. This is more than keyword matching—it involves reasoning about what the user wants to achieve.
- Planning Strategically: Once the goal is defined, the system breaks it down into smaller, manageable tasks. Advanced planning techniques, such as Monte Carlo Tree Search or Transformer-based decision models, help the agent choose the most effective path forward—almost like forming a mental roadmap.
- Remembering Context: Unlike traditional AI models that forget previous interactions, Agentic systems have memory. This could be long-term memory (like storing key facts) or episodic memory (like recalling recent conversations). These memories are stored using techniques such as vector embeddings, allowing the agent to bring up relevant information when needed.
- Taking Action: With a plan in place, the agent takes action—whether that’s calling an API, running a web search, or interacting with another system. It uses internal tools like scratchpads (temporary working spaces) or action queues to organize and execute its tasks in sequence.
- Learning from Feedback: Crucially, the agent doesn’t just act blindly. It observes the outcomes of its actions—either through system responses or human input—and updates its strategy if something didn’t work. This feedback loop enables continuous improvement and adaptability.
Working Together: Some frameworks, like CAMEL, show how multiple agents can work together or even hold conversations with humans to refine their understanding and collaborate toward shared goals. This simulates real teamwork—only it’s digital.
Working Together: Some frameworks, like CAMEL, show how multiple agents can work together or even hold conversations with humans to refine their understanding and collaborate toward shared goals. This simulates real teamwork—only it’s digital.
Generative vs. Agentic AI: What’s the Real Difference?
While Generative AI has revolutionized how we create content—whether it’s drafting text, designing visuals, or composing music—Agentic AI introduces a fundamentally different paradigm. The key difference lies in autonomy and initiative. Generative systems rely on explicit prompts and predefined data, operating within the boundaries of what they’ve been trained on.
In contrast, Agentic AI systems are proactive, continuously learning from their environments and autonomously pursuing goals with minimal supervision. This comparison clarifies how Agentic AI moves from mere content synthesis to intelligent action and adaptive behavior.
Few Areas Where Agentic AI is Making a Real Impact.
Can Agentic AI Reinvent How Financial Institutions Operate?
Non-Banking Financial Companies (NBFCs) increasingly adopt Agentic AI to enhance agility, risk mitigation, and client personalization.
a. Autonomous Risk Monitoring and Adaptive Fraud Detection
Agentic agents ingest transactional data streams and proactively detect outliers using reinforcement learning and continual memory updates. These systems adapt to evolving fraud patterns instead of relying on fixed rules. A case study from a Southeast Asian NBFC implemented an agent-based model leveraging time-series anomaly detection, reducing fraud losses by 25% year-over-year.
b. Dynamic Credit Scoring and Underwriting
Unlike static credit scoring models, Agentic systems incorporate dynamic behavioral features—such as recurring expenditure trends, social behavior scores, and even micro-market shocks to produce adaptive credit risk assessments. These models often use a mix of transformer-based learning and Gaussian process regression to personalize lending at scale.
c. Compliance Agents for Regulatory Intelligence
Agentic frameworks are being piloted to autonomously review policy changes and suggest system-level regulatory updates, reducing legal risk. Integrations with NLP systems and policy ontologies allow for accurate interpretation of financial mandates, streamlining compliance audits.
d. End-to-End Agentic Orchestration in Back-Office Operations
Drawing from a structured Agentic AI framework, NBFCs can integrate agents across the credit lifecycle—from underwriting to collections and compliance. For instance, an underwriting agent may recall historical risk outcomes, plan decisions using policy-aligned reasoning, and interact with internal APIs (e.g., fraud analytics, bureau reports). These agents then act autonomously while recording each decision for auditability. Meanwhile, compliance agents continuously scan regulatory updates and transaction logs to flag risks in real-time. As outcomes are recorded, feedback loops update memory, improving agent performance over time. This orchestration not only improves speed and accuracy but ensures that each decision is context-aware and self-correcting, reducing manual burden and enhancing regulatory confidence.
How Is Agentic AI Transforming Agriculture from Soil to Shelf?
Agentic AI is redefining how agricultural operations are managed—bringing a layer of intelligent decision-making to a traditionally intuition-based industry. By leveraging autonomous agents, farms and agribusinesses are enhancing resilience, optimizing yield, and reducing waste across the value chain.
a. Precision Farming and Resource Optimization
Agentic systems integrate data from soil sensors, drones, and weather APIs to autonomously guide irrigation schedules, nutrient deployment, and sowing cycles. One example includes a wheat farm in Australia using multi-agent coordination to calibrate nitrogen application, leading to over 20% higher yields with reduced input costs.
b. Autonomous Greenhouse Operations
Modern hydroponic greenhouses are adopting Agentic agents to control environmental variables like light, humidity, and nutrient flow. A vertical farming initiative in the Netherlands successfully applied these agents to optimize growth cycles and energy use, reducing operational costs by 30% while enhancing crop uniformity.
c. Proactive Pest and Disease Management
Advanced Agentic systems now analyze real-time plant imagery and compare it with disease profiles to trigger timely interventions. A tomato farming operation in India utilized this to detect fungal threats early, curbing the spread and saving a significant portion of the yield.
d. Supply Chain and Market Intelligence
In post-harvest logistics, Agentic agents are orchestrating cold chain routing and inventory management. For instance, a citrus exporter in North Africa deployed these agents to dynamically reroute shipments facing transport delays, reducing spoilage-related losses by nearly 18%.
These case studies demonstrate how Agentic AI not only handles discrete agricultural tasks but also manages entire operational workflows—combining environmental perception, historical learning, and real-time planning to drive productivity and sustainability.
A New Era of Intelligent Autonomy
Agentic AI marks a significant milestone in the evolution of automation—pushing beyond static task execution into the realm of intelligent, adaptive, and goal-driven systems. In sectors like finance and agriculture, its impact is already tangible, from autonomous credit underwriting to predictive crop management.
Yet with great power comes new responsibility. The very traits that make Agentic AI effective—independence, contextual learning, and dynamic decision-making also introduce challenges. Misaligned goals, opaque reasoning, and unchecked biases can create risk if oversight is neglected. That’s why successful deployment must blend innovation with intentional design, ethics, and monitoring.
Looking ahead, the future belongs to organizations that not only embrace Agentic AI’s capabilities but also shape its trajectory responsibly. By pairing technical advancement with human values and clear governance, we can ensure these agents become true partners in progress—working with us, and not just for us.